Personalization has been around for a long time and many businesses have begun to use data about customers to drive targeted marketing; but personalization is changing, and we’re now at the cusp of the next generation: Deep Learning.
In the beginning, consumers were targeted when they browsed a website or viewed a TV show. As consumers started regularly consuming content online, businesses began using the data obtained from the behavior history of their customers to provide more relevant content. In recent years, personalization engines have begun to use an even more fine-grained approach than website-by-website tracking. Instead, companies have begun to perform deep segmentation of users based on a variety of factors with the ultimate goal of providing a customized experience for each user.
At the same time, businesses have also begun to develop increasingly sophisticated systems to generate recommendations with an increasingly rich set of attributes. In addition, as consumers move increasingly into wearable devices, businesses will need to listen and respond even more finely to fine-grained cues from consumers.
This article will give you a peek into the world of deep learning and will explain how to leverage it in your personalization strategy.
What is Deep Learning in Personalization?
Deep learning is the next generation of personalization. It’s based on applying deep neural networks to computational models that require the use of artificial intelligence (AI). Deep learning has been around for a long time and used in a variety of domains like image recognition, natural language processing, and so on but it’s now at the cusp of becoming pervasive (and important) because it offers businesses an incredible ability to create highly targeted personalized content.
The concept of deep learning is based on the idea that increased amounts of data allow businesses to make more precise predictions about their users. This starts by gathering as much data as possible, analyzing these pieces with advanced computational models (that require AI), and using what’s learned to segment users according to particular attributes like lifestyle or purchase habits. The business will then personalize its content and services based on the specific needs of each segment. The ultimate goal is to deliver services that are perfectly tailored to each individual user.
Session-based Recommendation
session-based recommendations are recommendations that are based on the user’s interaction within a specific time frame. The most popular method currently being used to generate these recommendations is for between-session requests. The recommendation can then be triggered on a periodic basis in order to maximize the possibility of a user remembering his/her intent and remembering why the recommendation was important.
Leveraging Both Audience and Transaction Data
One method for providing session-based recommendations involves leveraging both audience and transaction data.
One simple example of this is a mobile search advertisement that recommends a mobile payment service in close proximity to a mobile payment transaction. In this case, the advertisement provides both an audience profile (e.g., people who have made mobile payments recently) and a transactional context (e.g., people who have made mobile payments around sunset time).
Leverage Deep Learning by Using Cookies
One of the ways that companies have been able to leverage deep learning is by using cookies to track user behavior. Automatic checking of a user’s cookies can help determine their demographic, location, and browsing history. This enables a company to segment users based on attributes like lifestyle or purchase habits. This is done through the use of an algorithm that predicts how likely an individual would be to make specific types of purchases online based on the attributes within their browser cookie information.
One example might be an ad placed in front of an item sold by Amazon for customers who are identified as having previously looked at other items sold by Amazon. The ad will then show up in front-end ads (e.g., on the side of the page while browsing the internet).
Deep Learning by Tracking Fingerprints
The other way that companies have implemented deep learning is by tracking device fingerprints. The main advantage of this approach is that it’s not reliant on cookies being enabled in order for deep learning to take place. One example of this type of technology is Adobe’s Audience Manager, which uses machine learning to predict what customers are doing, where they are doing it, and what they are likely to do next based on their actions in the past.
Adobe’s Audience Manager
Adobe’s Audience Manager recommends content by using data collected from various sources, including web cookies, Adobe locations services, CRM data, and real-time events like store visits. Predictions can be made about interests (i.e., what is the probability that someone who browses is likely to be interested in this item?) and location.
This is one of the most simple approaches for implementing deep learning in personalization. It’s based on the idea that users are more likely to buy products that are related to what they’ve previously bought or are interested in. However, this approach has a level of risk as user interests and browsing history can change over time. Additionally, it can be difficult to provide content-specific recommendations based on those types of metrics because they don’t reflect a user’s intent (i.e., what do you know about me?).
Deep learning has been used for many different purposes, one of which is extracting features from unstructured data. For example, in the environmental field, there is a great demand for large-scale feature extraction and classification because of the need for more reliable and accurate models that can predict ecological responses to current or future conditions. One way that this can be done is by leveraging convolutional neural networks (CNNs) to provide classification accuracy along with easy-to-interpret features like soil moisture content and leaf index.
The main point here is that deep learning provides a powerful tool for extracting features from unstructured data but it’s important to understand how they work in order to effectively make use of them.
One of the key components in overcoming the challenges related to the low popularity and profitability of OTT services is through session-based recommendations. Recent literature has shown that session-based recommendations can significantly increase overall streaming hours. However, to be successful, it’s important for providers to take into account both personalization and diverse interests as well as long-term engagement. The main goal with this type of recommendation is to make sure that individualized suggestions are made based on a single session rather than assuming certain preferences based on their overall viewing history.
Deep learning has also been used in the field of music recommendation. One way that deep learning can be implemented to recommend music is by finding correlations between similar expressions. Oth
er approaches have looked at deeper characteristics of songs, such as tempo or rhythm patterns, which are not limited to a single genre. This content goes beyond just recommending artists who are similar in genre and instead looks at more abstract aspects of songs. One of the best examples of this is Pandora Radio that uses “personalized radio”. They work by initially training a model that predicts what songs you would like based on your music library. The more you use it, the more accurate their prediction becomes and the more it learns about your taste in music.
Conclusion
Deep learning can be implemented for all different types of real-time recommendation use cases where the goal is to provide a user with an experience that matches their specific needs. It has been used in many different types of recommendation use cases, such as music and business. One of the main benefits of deep learning is that it provides a powerful tool for extracting features from unstructured data.
Deep-learning techniques such as convolutional neural networks (CNNs) provide a powerful way to extract features from unstructured data and effectively make use of them. Overall, it is important to understand how deep learning can be implemented in order to effectively make use of it.
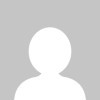
Leave a Reply