With the advent of technology and the global pandemic, the banking sector has evolved rapidly, and clients’ expectations have grown. A massive shift toward online platforms has raised contentions for a more satisfactory user interface. Given these modern technological transformations, Artificial Intelligence and Machine Learning in banking and financial services are gaining higher traction as banking institutions now understand the significance of automation for crucial procedures.
Approximately 85% of top-level industry decision-makers think AI in banking will add some meaning and advantages to their enterprise processes in the coming days. Keep reading below to learn more about the need for AI in banking, along with its existing obstacles and solutions.
Understanding the Role of AI in Banking
Even though AI in financial services and banking is a newly emerged concept, it is already on its way to making financial institutions more accessible, efficient and secure.
Furthermore, to leverage AI and deep learning in investment banking, financial institutions must continuously innovate to address the increased demand from modern tech-savvy clients and financial regulators. Hence it might not be wrong to say that adopting AI in the banking domain will transform money management in the coming years.
Top Advantages of Artificial Intelligence and Machine Learning in Banking?
Better investment evaluation
Interest earnings are only one aspect of revenue generation for banks and financial institutions. Therefore, they continually look for lucrative possibilities to invest and earn profitable returns. This is where AI comes into the picture. With the help of advanced AI-based investment software, banks can gain valuable investment recommendations that match their risk-taking capacity.
Furthermore, it can assist in accurately assessing customer funding proposals, given that industry-relevant data is often challenging to understand. However, you must note that the decision to invest funds remains in the hands of human beings. This investment analysis software makes the process more seamless and allows the decision-maker to accommodate more variables.
Reduced risks and operational costs
While people enjoy the physical feel and human interaction in banking, it has a considerable drawback. Manual errors are inevitable, and they can sometimes have severe repercussions.
In addition, even though trained employees usually make fewer manual mistakes, even one could expose the bank or financial institution to penalties and cause irreparable reputational harm. On the other hand, AI-based decision management systems lower this risk by making logic streams in the data capture and incorporating predictive and prescribed methods to solve enterprise issues.
Enhanced client experience
Modern clients are continually looking for ease and convenience. For instance, the ATM (Automated Teller Machine) facility was a hit because clients could access integral assistance even when banks and financial institutions were closed. That level of comfort has only encouraged more innovation.
Furthermore, with the help of AI in financial services, customers can now open a bank account with their web or mobile devices right from the comfort of their homes. Additionally, with appropriate business management software, financial decisions can be executed and rolled out without extensive procedures.
What Are Some Potential Use Cases of AI in Banking?
Artificial Intelligence and Machine Learning have become integral aspects of banks and financial institutions. They have started integrating AI technologies into their business operations to offer better convenience to their customers. Some of the possible AI banking use cases are as follows:
Investment analysis
In investment analysis, AI and ML help banking sectors make informed data-backed decisions. AI and ML algorithms can efficiently identify trends and relationships by deeply analyzing market prices, economic indicators, historical performance, and news sentiment.
With the power of deep learning, the AI and ML models not only assess the trends; they also can predict future market movements based on historical data. For instance, they can predict stock price movements, interest rate changes, and market volatility. These predictions can guide investment strategies and enable banks to position themselves advantageously.
Customer Onboarding and KYC Processes
Intelligent Document Processing is setting a benchmark in banking and insurance automation. The AI and ML models can effortlessly convert forms, reports, and other documents into digital data, making handling and processing of data easier. This potential of the AI and ML model can make the customer onboarding and KYC process a breeze.
Intelligent platforms like Docusense extract and sync the data to the centralized data system. Therefore, employees can easily access the information whenever required and also can use the data to fill out the applications and make customer onboarding quick. As a result, employees can quickly access information whenever they need it, and they can also use the data to fill out applications, verify KYC compliance, and speed up customer onboarding.
Loan and Credit decisions
AI and ML can help in evaluating the creditworthiness of a person in detail, which will come in handy for loan and credit decisions. The AI and ML model goes through key metrics like credit history, income, employment stability, and more to provide an accurate and comprehensive credit score.
With in-depth analysis, banks can quickly identify potential clients, provide personalized loan offers, and improve conversion rates.
Regulatory compliance
Regulatory compliance is a pivotal point in every banking process. Maintaining and following regulatory compliances can be made simple with the AI and ML automation solution. Whether it is a loan processing or KYC verifications, the AI model quickly goes through the historical datasets and checks if there is any anomaly in the process according to the law and other compliance acts.
Apart from verifying and flagging the reports, some AI and ML models regularly track for any change in the regulations. With automated and up-to-date regulatory change management, the banks can ensure compliance management throughout the year.
Information retrieval and content summarizer
Information retrieval from sources is the core characteristic of any AI and ML model. With Advanced NLP and computer vision technology, the AI can extract accurate information from sources like structured documents, images, handwritten copies, unstructured documents, and many more.
When entity recognition is also combined with the information retrieval process, the AI platform can effortlessly extract the information and classify it as per the set entity parameters (for example, names, dates, and locations).
Content summarizing is smarter and a grade higher than the information retrieval process. The AI and ML solution can identify the most important sentences or passages in a banking/financial document and extract them to create a concise summary while maintaining the original context. The summaries can be filtered and can also be clustered for easier navigation and quick access.
Fraud detection in money lending
Artificial intelligence (AI) and machine learning (ML) technologies are transforming fraud detection in the financial sector. They use sophisticated techniques such as anomaly detection and predictive modeling to analyze transaction data for unusual patterns and predict potential fraudulent activity. These technologies provide real-time monitoring across multiple channels, creating a comprehensive view of transactions and behaviors.
ML models continuously learn from historical cases, adapting to evolving fraud tactics. Additionally, they provide geolocation analysis and network examination to uncover complex fraud networks. By enhancing security and minimizing false positives, AI and ML ensure regulatory compliance, protecting financial institutions and their customers.
AI Adoption: Obstacles and Solutions
Here are some prominent obstacles in AI adoption and its possible solutions:
Explainability, Clarity and Trust
Developing AI models that deliver precise projections can sometimes be challenging for banks and financial institutions. Furthermore, it can also be time-consuming for senior management to explain advanced AI-based applications to their employees. Nevertheless, this issue can get resolved with adequate training and the development of easy data models.
Black Box Impact
The intricacy of neural devices makes Machine Learning and artificially intelligent software challenging to interpret. Hence to overcome this challenge, financial institutions and banks must increase governance and embrace visual understanding and model administration frameworks.
The Bottom Line
In a nutshell, Artificial Intelligence and banking go hand in hand, given the countless advantages this modern technology offers. In addition, according to a report, 65% of senior banking and financial management anticipate favourable transformations from adopting AI and Machine Learning in the finance and banking domain. Hence, it might not be wrong to say that every financial institution must invest in the latest AI technologies to offer exceptional client services and make operations more seamless.
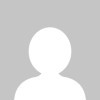
Leave a Reply